Breadcrumb
Online tracking: Iowa investigators use search history data to predict Lyme disease rates
Published on March 10, 2022
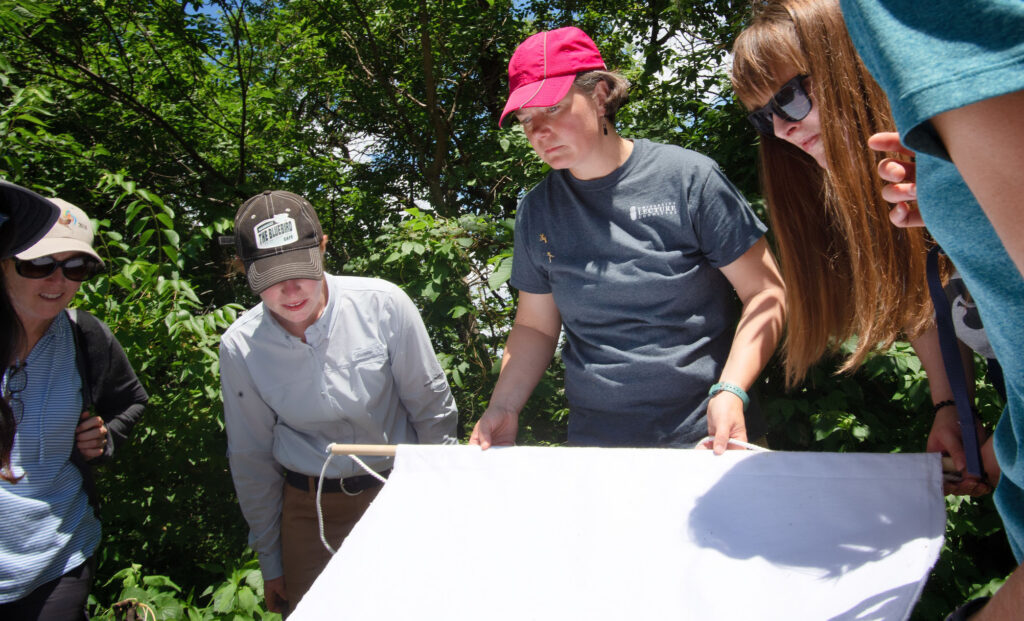
While winter-weary Iowans anxiously await the arrival of warmer temperatures and more frequent outdoor activities, the change of seasons also marks the reappearance of a disease-carrying pest that can spoil any springtime celebration: the Ixodes tick.
Commonly known as the black-legged tick or deer tick, Ixodes species begin searching for meals — blood meals — during spring and early summer months, and humans venturing outside for work or recreation are on the menu. A bite by one of these infected arachnids can transmit a variety of pathogens including Lyme disease, the most widely reported vector-borne disease in the United States.
To help protect against these hungry and often nearly invisible ectoparasites, a team of University of Iowa epidemiologists has developed new disease surveillance strategies that couple historical data with information drawn from internet search terms to predict current trends in Lyme disease. The researchers say their innovative models are tools that local health agencies can use to tap free, up-to-date information — in this case, search history data from Google — to better understand Lyme disease patterns and make more timely, targeted interventions to battle the steadily rising incidence of the disease.
The UI team, led by Professor of Epidemiology Christine Petersen, set out to develop these “nowcasting” models — so-called because they’re designed to “predict the present” — to address reporting lags that hamper current public health surveillance and mitigation efforts. Their research was recently published in the open-access journal PLOS ONE.
“Optimal surveillance of Lyme disease requires reporting from multiple parties,” says first author Eric Kontowicz, who earned his PhD in epidemiology from the University of Iowa in 2020 and now is a postdoctoral research associate at Purdue University. He notes that case reports originating from individual physicians, public health laboratories, and hospital laboratories are collated at the state level, then submitted to the U.S. Centers for Disease Control and Prevention (CDC), which ultimately reports out federal trends.
“This process can create a close to two-year lag in nationwide maps and reports from the CDC. Local and state health departments have to predict current and emerging public health needs in their community, and often this data is too late to be useful.”
Lyme disease facts:
- Each year, approximately 30,000 confirmed Lyme disease cases are reported nationwide, with about 95 percent of cases occurring in the Upper Midwest and Northeast regions of the U.S. Cases in Iowa have increased 20-fold in the last decade.
- Typical symptoms of Lyme disease include fever, headache, fatigue, and a characteristic “bulls-eye” skin rash called erythema migrans. If left untreated, infection can spread to joints, the heart, and the nervous system.
- Due to differences in reporting Lyme disease data from states and localities, compilation of data at the federal level can take several years.
The research team built statistical models capable of predicting Lyme disease incidence in five regions of the United States: Northeast, Midwest, Southeast, Southwest, and West. Two different models were developed for each geographic region — one model using only search terms related to the name, symptoms, and ticks that carry Lyme disease, and another model using those same disease-specific terms plus a broader list of terms, identified by Google Correlate, that an average person would be searching for during internet browsing. The researchers reviewed internet search data between 2004 and 2019, collected at monthly intervals to match against Lyme disease incidence data from the CDC.
When fit to the CDC data, the researchers found that both models provided accurate estimates of Lyme disease incidence in four of the five geographic regions; however, the model that included colloquial search terms resulted in more accurate predictions. Including the list of expanded search terms produced predictions that had a 1.33-fold improvement in accuracy and 0.5-fold reduction in error compared to the symptom and tick terms-only models.
Many of the terms in the full list found to be important for model performance were environmentally themed, suggesting intent to take part in outdoor activities such as concerts, camping, and water parks — places where people are likely to be exposed to Ixodes ticks during the late spring, summer, and early fall. Predictions from the full-list models also produced accurate timing of seasonal patterns of Lyme disease and improved mimicking of peaks and recessions.
“In order to be exposed to ticks we must travel into their environments,” says Petersen. “Including terms related to camping, hiking, and the other summer activities allowed for some proxy measure of individual’s intentions to spend time in environments where Borrelia infected ticks live.”
Increasing internet usage has changed the way individuals seek and receive health information, Kontowicz says. These changes provide researchers with new opportunities to improve disease prediction and public information.
“Using web-based data from Google or social media sites to predict health outcomes is gaining popularity and credibility from a variety of public health audiences,” observes Kontowicz. “These non-traditional indicators of disease spread or exposure allow researchers to include some level of human behavior or intention into their modeling efforts.
“This data is readily accessible and may help fill in gaps in times of reporting lag, but we still need strong surveillance efforts and data to ensure that these models and predictions will be accurate. This combination of strong surveillance efforts and data coupled with computational modeling techniques can generate models that produce accurate predictions of disease trends.”
The study, “Inclusion of environmentally themed search terms improves Elastic Net regression nowcasts of regional Lyme disease rates,” was published March 10, 2022. In addition to Petersen and Kontowicz, the UI study team included Grant Brown, assistant professor of biostatistics; Jim Torner, professor of epidemiology; Margaret Carrel, associate professor of geographical and sustainability sciences; and Kelly Baker, assistant professor of occupational and environmental health.